Personalized product recommendations are revolutionizing the way we shop online. By tailoring suggestions to individual customers, businesses can provide a personalized shopping experience that improves customer engagement and drives sales. Companies like Amazon and Netflix have already harnessed the power of personalized recommendations, and their success speaks for itself.
However, while the benefits of personalized recommendations are undeniable, there are hidden costs that you may not be aware of. In order to deliver tailored suggestions, businesses rely on user data, including browsing history and preferences. This data is used to fuel recommendation algorithms and create a more customized shopping experience.
But what does this mean for you as a customer? It means that the choices you make, the products you view, and the information you provide are all being collected and analyzed. Your data is valuable, and companies are willing to invest in personalized recommendation engines to access it. So while you may enjoy the convenience and curated options, there is a cost – your personal data.
It’s important to consider the trade-off between the benefits of personalized recommendations and the privacy of your data. Are you comfortable with companies using your personal information to make suggestions? Are you willing to sacrifice some privacy for a more tailored shopping experience? These are questions that every customer should ask themselves before embracing personalized recommendations.
Key Takeaways:
- Personalized recommendations offer a tailored shopping experience and drive sales
- Implementing personalized recommendations relies on user data and preferences
- There is a trade-off between the benefits of personalized recommendations and the privacy of your data
- Consider whether you are comfortable with companies using your personal information to make suggestions
- Weigh the benefits and costs of personalized recommendations before embracing them
What are Personalized Product Recommendations?
Personalized product recommendations are a game-changer in the world of online shopping. By tailoring content and suggestions to individual users, businesses can offer a more customized and seamless shopping experience. These recommendations are based on customers’ preferences, browsing history, and behavior, allowing businesses to curate a selection of products that are most likely to appeal to each customer.
Personalized product recommendations revolutionize the way customers interact with online stores. Instead of overwhelming users with countless options, these tailored recommendations narrow down the choices and present customers with products that align with their interests and needs. Whether it’s suggesting best-selling items, frequently bought together products, or trending products, these recommendations enhance the user experience by providing relevant and enticing suggestions.
For example, imagine a customer browsing an online fashion retailer’s website. Personalized recommendations would consider the customer’s browsing history and past purchases to suggest items that match their style preferences. By doing so, the retailer not only simplifies the customer’s decision-making process but also increases the likelihood of a purchase being made.
Benefits of Personalized Product Recommendations: |
---|
1. Enhanced user experience through tailored suggestions |
2. Increased customer engagement by narrowing down choices |
3. Improved conversion rates and higher sales |
4. Reduced decision fatigue and choice overload |
5. Encouragement of impulse purchases through strategic recommendations |
Personalized product recommendations not only benefit customers but also provide a significant advantage to businesses. By offering tailored suggestions, businesses can boost their sales, improve customer satisfaction, and increase customer loyalty. It’s a win-win situation that optimizes both the shopping experience and a business’s bottom line.
Now that we understand the concept and benefits of personalized product recommendations, let’s explore how these recommendations actually work.
How Do Product Recommendation Engines Work?
Product recommendation engines play a crucial role in enhancing the personalized shopping experience for customers. These engines utilize sophisticated algorithms to analyze user data and generate tailored recommendations. By analyzing factors such as search queries, product views, shopping history, and geographic location, these engines can provide personalized recommendations that cater to individual preferences and interests.
There are different types of recommendation filtering systems employed by these engines. Collaborative filtering is one such approach that focuses on analyzing user interactions and preferences. By examining the behavior of similar users, collaborative filtering generates recommendations that align with the user’s interests.
Content-based filtering, on the other hand, concentrates on the characteristics and attributes of items. By identifying the key features of products, content-based filtering can suggest items that are similar or complementary to what the user has previously shown interest in. This approach is particularly effective in situations where user preferences may not be explicitly expressed through past interactions.
Hybrid filtering is a combination of collaborative and content-based approaches. It incorporates both user behavior and item attributes to generate more accurate and diverse recommendations. By leveraging a hybrid model, product recommendation engines can provide a comprehensive and highly personalized shopping experience.
The Types of Product Recommendation Filtering:
- Collaborative Filtering
- Content-based Filtering
- Hybrid Filtering
As an example, let’s say a user frequently purchases athletic shoes and also regularly visits product pages related to fitness equipment. A collaborative filtering recommendation engine would analyze the behavior and preferences of other users who have demonstrated similar patterns. It might then suggest items such as running shorts, sports watches, or workout accessories based on what this particular user group tends to buy.
On the other hand, a content-based filtering recommendation engine would analyze the characteristics and attributes of the products the individual has shown interest in. It might recommend other athletic shoe brands or fitness equipment with similar features that align with the user’s preferences.
By using a hybrid filtering approach, the recommendation engine can combine the insights gained from both collaborative and content-based filtering to provide even more accurate and diverse recommendations. This ensures that customers receive tailored suggestions that align with their individual tastes, leading to an enhanced shopping experience and increased customer satisfaction.
Collaborative Filtering | Content-based Filtering | Hybrid Filtering |
---|---|---|
Analyzes user interactions and preferences | Focuses on the characteristics of items | Combines both collaborative and content-based approaches |
Recommends items based on the behaviors and preferences of similar users | Suggests items based on the attributes and features of products | Provides more accurate and diverse recommendations |
Uses the collective wisdom of similar users to generate suggestions | Identifies items with similar features to those previously shown interest in | Offers a comprehensive and highly personalized shopping experience |
Types of Product Recommendations Filtering
In the world of personalized product recommendations, there are three main types of filtering systems: collaborative filtering, content-based filtering, and hybrid filtering. Each of these approaches plays a crucial role in enhancing the customer shopping experience by providing tailored suggestions based on different criteria.
- Collaborative Filtering: Collaborative filtering utilizes the preferences and behaviors of similar users to make recommendations. By analyzing data from like-minded shoppers, this filtering method identifies patterns and makes suggestions based on common interests. For example, if you’ve enjoyed products that other customers with similar tastes have also liked, collaborative filtering would recommend similar items to you.
- Content-Based Filtering: Content-based filtering, on the other hand, focuses on the characteristics and attributes of products themselves. By analyzing the features, descriptions, and metadata of items, this method recommends products that share similar qualities. For instance, if you’ve shown interest in a particular genre of movies, content-based filtering would suggest other movies within the same genre.
- Hybrid Filtering: Hybrid filtering combines the power of both collaborative filtering and content-based filtering to provide more precise and varied recommendations. By leveraging the strengths of both approaches, hybrid filtering offers a comprehensive solution that takes into account both user preferences and product attributes. With this method, customers can discover new items while still receiving suggestions that align with their personal tastes.
Each filtering system has its advantages, and businesses can choose the one that best suits their needs and goals. Collaborative filtering allows for personalized recommendations based on user behavior, while content-based filtering focuses on the inherent qualities of products. Meanwhile, hybrid filtering offers a balanced approach that enhances the overall customer experience.
Implementing the right recommendation filtering system can significantly optimize the shopping experience for customers, leading to increased engagement, satisfaction, and ultimately, higher sales conversions.
Filtering System | Advantages |
---|---|
Collaborative Filtering | – Leverages user preferences – Identifies patterns and similarities among users – Provides personalized recommendations based on user behavior |
Content-Based Filtering | – Focuses on product characteristics and attributes – Recommends items with similar qualities – Helps users discover new products within their interests |
Hybrid Filtering | – Combines collaborative and content-based approaches – Offers more precise and varied recommendations – Enhances overall customer experience |
Benefits Recommendations Engines Have to Offer
Implementing personalized product recommendations on a website or app offers several benefits. These recommendation engines are designed to provide tailored suggestions to customers, improving their shopping experience and driving revenue for businesses.
Improved Average Order Value (AOV)
Personalized recommendations can significantly impact the average order value (AOV) by suggesting complementary products to customers. By suggesting items that are relevant to their interests or previous purchases, recommendation engines can increase the value of each transaction.
Enhanced User Engagement
Personalized recommendations keep visitors engaged on the site, reducing bounce rates. By providing curated suggestions that align with users’ preferences and browsing behavior, recommendation engines enhance user engagement and encourage them to explore more products and content.
Higher Conversions and Reduced Cart Abandonment
Recommendation engines play a crucial role in increasing conversions. By suggesting relevant products, customers are more likely to make a purchase, resulting in higher conversion rates. These engines also help reduce cart abandonment by reminding users of items they previously showed interest in, encouraging them to complete their purchase.
Optimized Inventory
By analyzing customer preferences and purchase patterns, recommendation engines provide insights that allow businesses to optimize their inventory. This helps in effectively managing stock levels, reducing inventory costs, and improving overall efficiency.
Time-Saving Automation
Recommendation engines automate the process of setting up upsells and cross-sells, saving time for businesses. By leveraging algorithms and customer data, these engines generate personalized recommendations at scale, eliminating the need for manual selection and setup.
Overall, the implementation of recommendation engines brings numerous benefits to businesses, including improved average order value, enhanced user engagement, higher conversions, reduced cart abandonment, optimized inventory, and time-saving automation.
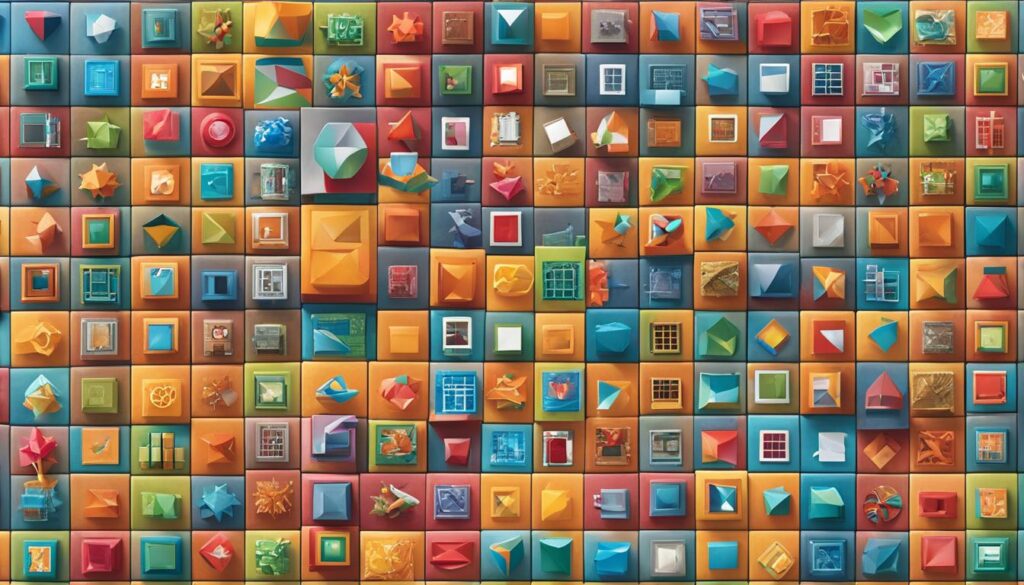
Reactions and Expectations of Users Towards Personalized Recommendations
Users generally have positive reactions to personalized recommendations, appreciating the curated suggestions tailored to their interests. These recommendations help users avoid choice overload and discover new items or content.
“I love how personalized recommendations save me time and help me discover new products that I wouldn’t have found on my own.”
While some users are concerned about privacy, most are willing to sacrifice some privacy for the benefits of personalized recommendations.
“I understand that my data needs to be collected to provide personalized recommendations, and as long as my privacy is respected, I’m okay with it.”
Users expect that their browsing history, purchase history, and other data will be used to generate recommendations. They also assume that algorithms and similar user patterns are taken into account when generating suggestions.
“I expect that the system will analyze my past interactions and preferences to provide me with relevant recommendations.”
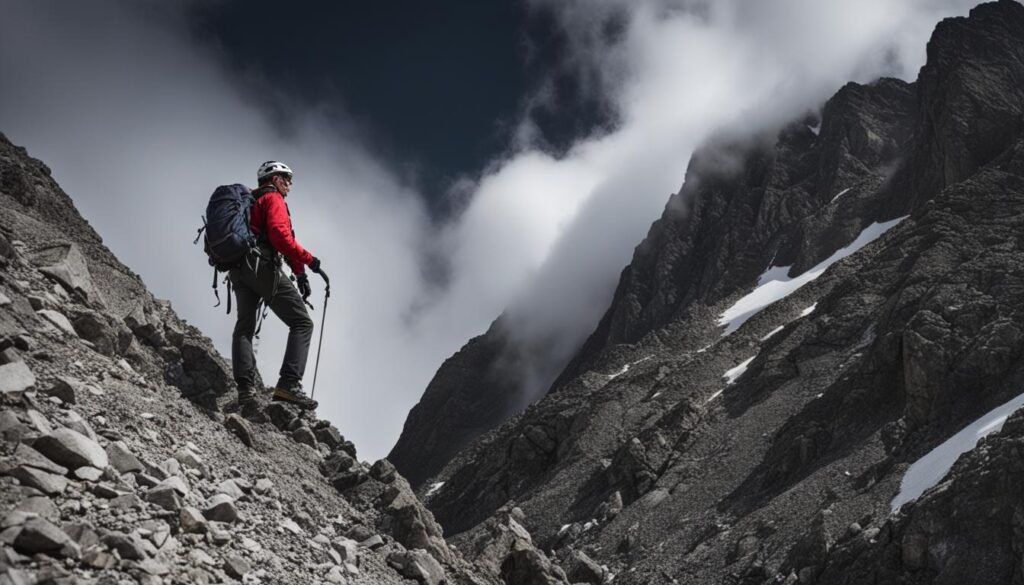
Overall, users appreciate the convenience and value of personalized recommendations and understand the trade-off between privacy and enhanced shopping experiences.
Judging Generic vs. Personalized Content
When users visit a website or app, they often look for signs that indicate whether the content is personalized or generic. These indicators help users determine if the recommendations and suggestions align with their preferences and needs. Several factors contribute to the judgment of content, including headings, explicit customization instructions, overall popularity, business motivations, and the likelihood of customization.
Headings: Clear markers like “Recommended For You” or “Because You Watched” are obvious indicators of personalized content. These headings create an expectation that the recommendations provided are tailored to the individual user, based on their previous behavior and preferences.
Explicit customization instructions: If explicit instructions are given, such as “Tell us about your interests” or “Customize your feed,” users understand that the content will be personalized once they provide the necessary information. These instructions give users a sense of control over the recommendations they receive, increasing the perceived relevance of the content.
Popularity: When content is popular among a large number of users, it can be perceived as generic rather than personalized. Users assume that personalized content will cater to their unique tastes and preferences. If a recommendation algorithm suggests the same popular items to every user, it may be seen as less personalized.
Business motivations and the likelihood of customization: Users consider the motivations of the business behind the content. If a company heavily emphasizes personalization in its marketing messages and promises tailored recommendations, users are more likely to expect a high level of customization. On the other hand, if a company’s primary goal is to promote specific products or maximize profits, users may doubt the level of personalization.
Structure and design: The structure and design of the service also play a role in shaping user expectations and perceptions of personalized content. A well-designed interface with intuitive customization options can enhance the perception of personalization, while a cluttered or confusing layout may diminish trust in the content’s relevance to the user’s preferences.
Factors | Indicators |
---|---|
Headings | “Recommended For You,” “Because You Watched” |
Explicit customization instructions | “Tell us about your interests,” “Customize your feed” |
Popularity | Perceived as generic |
Business motivations and likelihood of customization | Marketing messages, profit-driven strategies |
Structure and design | Intuitive interface, cluttered layout |
Ultimately, judging content as generic or personalized is a subjective process for users. They rely on various cues and contextual factors to determine the level of customization in the recommendations and suggestions they encounter. By understanding these indicators, businesses can optimize their content to meet user expectations and deliver truly personalized experiences.
Can Personalized Recommendations and Diversification Strategies Work Together for Better Results?
Yes, personalized recommendations and diversification strategies insights and tips can definitely work together for better results. By understanding individual preferences and utilizing diversification in investment or product offerings, companies can provide a more tailored and comprehensive experience for their users or customers.
Conclusion
Personalized recommendations have the power to revolutionize the user experience by providing tailored suggestions that cater to individual preferences. Implementing recommendation engines can bring numerous benefits to businesses, including increased revenue, improved average order value (AOV), higher conversions, and reduced cart abandonment. Users generally have positive reactions to personalized recommendations and are willing to compromise on their privacy in exchange for the advantages they bring.
By understanding user expectations and leveraging the potential of personalized recommendations, businesses can make informed decisions to optimize their offerings and enhance customer satisfaction. These recommendations not only save time and effort for users by offering curated suggestions but also help them navigate through an overwhelming range of choices. Furthermore, the insights gained from user data enable businesses to provide more relevant and engaging experiences, leading to better customer engagement and loyalty.
Overall, the implementation of personalized recommendations benefits both businesses and users. Businesses can increase their revenue and customer satisfaction, while users can enjoy a more personalized shopping experience. By embracing the power of personalized recommendations, businesses can enhance user engagement, improve conversions, and meet the evolving needs and expectations of their customers in a data-driven world.
FAQ
What are personalized product recommendations?
Personalized product recommendations involve tailoring content and suggestions to individual users based on their preferences, browsing history, and behavior. These recommendations provide relevant and curated suggestions, narrowing down the options available on a website or app.
How do product recommendation engines work?
Product recommendation engines use algorithms to personalize the shopping experience for customers. They analyze user data such as search queries, product views, shopping history, and geographic location to generate personalized recommendations. Different types of recommendation filtering systems, including collaborative filtering, content-based filtering, and hybrid filtering, are used to offer accurate and diverse recommendations.
What are the different types of product recommendation filtering?
There are three main types of product recommendation filtering: collaborative filtering, content-based filtering, and hybrid filtering. Collaborative filtering recommends products based on the preferences and behaviors of other similar users. Content-based filtering suggests items based on the characteristics and attributes of the items themselves. Hybrid filtering combines both approaches to provide more precise and varied recommendations.
What are the benefits of recommendation engines?
Implementing personalized product recommendations on a website or app offers several benefits. It can improve the average order value by suggesting complementary products to customers. Personalized recommendations also enhance user engagement, leading to higher conversions and reduced cart abandonment. Recommendation engines provide insights into customer preferences, helping businesses optimize their inventory. They also save time by automating the process of setting up upsells and cross-sells.
How do users react to personalized recommendations?
Users generally have positive reactions to personalized recommendations, appreciating the curated suggestions tailored to their interests. These recommendations help users avoid choice overload and discover new items or content. While some users are concerned about privacy, most are willing to sacrifice some privacy for the benefits of personalized recommendations. Users expect that their browsing history, purchase history, and other data will be used to generate recommendations.
How do users judge whether content is personalized or generic?
Users rely on indicators such as headings, explicit customization instructions, and overall popularity to determine whether content is personalized or generic. Clear markers like “Recommended For You” or “Because You Watched” are obvious indicators of personalized content. Users also consider business motivations and the likelihood of customization when judging whether content is tailored to their preferences.
What is the conclusion?
Personalized recommendations can greatly enhance the user experience by providing curated suggestions tailored to individual preferences. Businesses can benefit from implementing recommendation engines, which lead to increased revenue, improved average order value, higher conversions, and reduced cart abandonment. Users have positive reactions to personalized recommendations and are willing to sacrifice some privacy for the benefits they bring. By understanding user expectations and leveraging the power of personalized recommendations, businesses can make informed decisions on how to optimize their offerings and improve customer satisfaction.